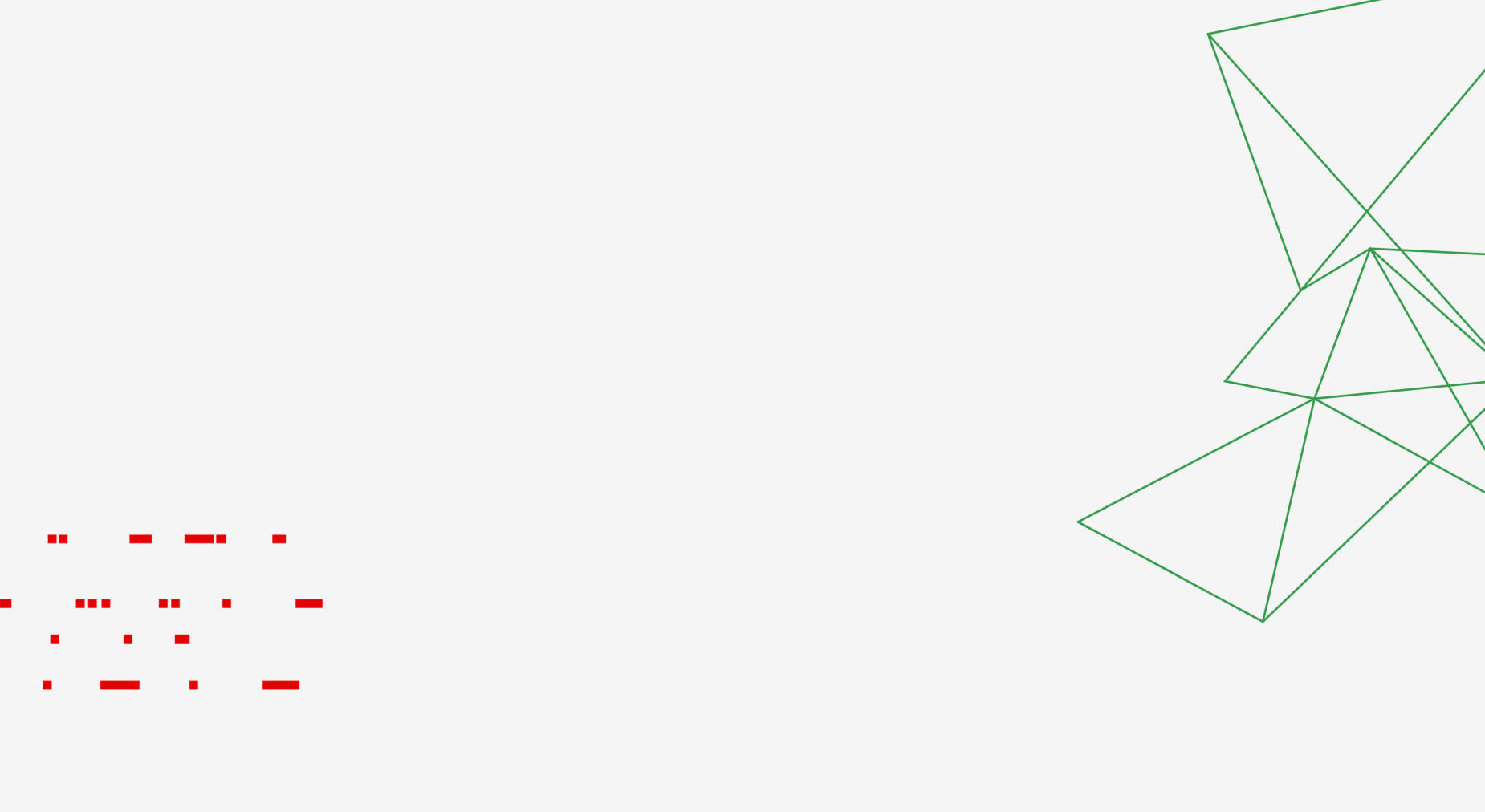
Handlungsempfehlungen für die Zukunft der Künstlichen Intelligenz
Um einen Beitrag zur Debatte über die Verbesserung der globalen KI-Governance zu leisten, veranstalteten wir beim Paris Peace Forum 2021 einen digitalen Vorausschau-Workshop. Die Teilnehmenden setzten sich mit zukünftigen Trends der globalen KI-Governance in diversen Politikbereichen auseinander. Dazu gehörten Gesundheitswesen, kritische Infrastruktur, Grenzmanagement, Wahlen und autonome Waffen. Dabei wurden Entwicklungen im Kontext von Datenschutz, Cybersicherheit, globalen Ungleichheiten und geopolitischen Auswirkungen neuer Technologien als Querschnittsthemen mitgedacht.
Multistakeholder engagement and cooperation is essential to robust AI governance now and will continue to be so in the future. Effectively governing digital global goods cannot be achieved by states alone but depends upon various actors joining forces. But all engagement is not created equally: success requires meaningful participation by all material stakeholders on the issue under consideration. In structuring such fora, decision-makers should consider three things.
First, they should capitalize on existing channels. To avoid diverting precious time and resources, policymakers should prioritize AI governance concerns in organizations and efforts in which they already participate, rather than devoting time and resources to establishing new platforms for engagement, like the World Truth Council. One strong existing example of this is the Globalpolicy.ai initiative, through which a number of intergovernmental organizations with AI mandates are collaborating to share information and resources.
Second, they should take robust, respectful inclusion seriously. Just as policymakers take advantage of existing networks, it is crucial to consider voices that are not yet adequately represented in the AI governance conversation. This is particularly apposite as regards historically marginalized communities, with whom some policymakers may not have strong existing relationships. Stakeholder outreach should be conducted respectfully, avoiding tokenism and remaining mindful of any interests the relevant communities have already communicated through organizations like the Indigenous Protocol and Artificial Intelligence Working Group.
Finally, timing is crucial. Multistakeholder cooperation may be advantageous throughout the development and implementation of policy solutions, from identifying and prioritizing issues through deployments and later amendments. Consultations should be structured appropriately for each stage of the process to ensure inputs that are offered can be thoroughly considered and addressed.
A high degree of transparency about the processes leading to decisions is essential for trust in AI systems. The degree of required transparency is contingent on the use-case and the severity of the consequences of a mistake. But understanding a decision is also highly context-dependent. An explanation suitable for an expert in machine learning may be unsuitable for the implementing domain expert (e.g. an immigration or customs official) or the impacted layperson. Information that is sufficient for achieving understanding in an urgent setting (e.g. a medical emergency) may be insufficient in another (e.g. avoiding discrimination in a border management system). For this reason, policymakers should expect providers and users of high-risk AI systems to produce information that meets the needs of multiple audiences, may vary depending on the situation, and enables scrutiny across multiple dimensions. Some of the most advanced AI and machine learning techniques do not produce interpretable explanations for the generation of a particular decision. Whether such systems should be used in high-risk settings at all is a matter of ongoing public debate. The European Commission’s proposed AI Act requires high-risk AI systems to enable users and human overseers to interpret their outputs. It is still unclear if this requirement categorically excludes the use of uninterpretable techniques, or if the construction of post-hoc models that explain the behavior of the underlying model will be accepted as sufficient. As AI systems are potentially beneficial to citizens in high-stakes contexts, policymakers should carefully consider whether the benefits of utilizing techniques that are not fully interpretable outweigh the risks in some instances.
The digital economy uses interconnected data in ways that often have societal effects, but data is governed as though it were a solely personal matter. It is the aggregation of data and its connection to other data that enables new insights and predictions to be made with artificial intelligence, machine learning, and statistical models. The laws and regulations that control the capture and use of data preserve the rights of individual data subjects, address direct or indirect privacy or other harms that have accrued to individuals, and mediate the relationship between data subjects and data processors. But the societal impacts of these technologies are often diffuse, occurring outside the boundaries of the relationship between the data subject and the data controller. Crucially, tremendous value is derived from the relationships of data to other data: observations from a small sample enable inferences about whole populations, the addition of new data from others can yield insights about a data subject that they themselves were unaware of, and data voluntarily provided by an individual may impact the interests of another person who is not a data subject. Going beyond individual protections and addressing the cumulative effects of the data economy is a crucial challenge for the current generation of policymakers. Governments, companies, and citizens have already begun to develop alternatives to top-down data governance models through data cooperatives, trusts, and other mechanisms. These nascent efforts vary in form and purpose, but enable greater participation by individuals and groups in decisions concerning their data. Policymakers should invest in supporting such efforts within their regions or subject area remits in order to foster a rich ecosystem of complementary approaches to data governance. Promising avenues include supporting academic research in this area, establishing regulatory sandboxes, and collaborating internationally on the development of technical and governance mechanisms.
As regulatory bodies across the globe begin to take on the challenge of AI, procurement is a natural starting point and a powerful lever for policymakers to pull. Governments possess considerable purchasing power, which translates into influence. Numerous jurisdictions, beginning with Canada in 2019, have already sought to employ procurement regulations in the governance of AI technologies. There is robust guidance available from the World Economic Forum and the European Union, and further information may be available through the OECD.AI Policy Observatory. One advantage of procurement as a regulatory strategy is that it is equally viable for regional and municipal government entities. In structuring AI procurement policies, decision-makers should consider at least three things. First, the public sector has considerable standard-setting power. It can thus model best practices for private entities purchasing similar systems, such as conducting impact assessments prior to acquiring an AI-based product. Second, government procurement policies shape products; and they can influence the market for AI solutions in several ways. For example, they can be an engine of transparency. Canada’s regulation requires the government to publish custom source code it acquires; this supports auditability and increases the knowledge base available to advance innovation. More generally, if governments use their purchasing power to insist on rights-protective, responsible technological solutions, vendors are incentivized to build products that comply with those requirements, and other downstream buyers benefit from the same changes. Lastly, public actors should be mindful of their broader influence on other stakeholders. As policymakers begin to see domestic benefits from responsible AI procurement policies, they may also consider encouraging allies and partners to adopt them as well, for instance in the context of regional fora, negotiations, and trade agreements.